Network Medicine for Discovering Gene Modules and Precision Medicine in ME/CFS
The pathophysiology of ME/CFS is unknown and no effective treatment is currently available for the disease. The number of omics studies of ME/CFS increases rapidly, but due to underfunding on this disease, most studies are of small sample size and a single type of measurements. In addition, while sharing core symptoms, different groups of patients suffer distinctive sets of dominant symptoms. There is an urgent need to effectively integrate studies of ME/CFS along with other well-studied diseases to discover the molecular mechanism(s) of ME/CFS and to identify gene/pathway targets. This proposed project will apply deep-learning based network medicine approaches to study ME/CFS for discovering the disease modules and repurposing drugs for ME/CFS. A focus is also on precision medicine in ME/CFS, to identify tailored treatment strategies for specific debilitating symptoms in patients, e.g. fatigue vs sleep problems vs pain vs multiple sensitivity.
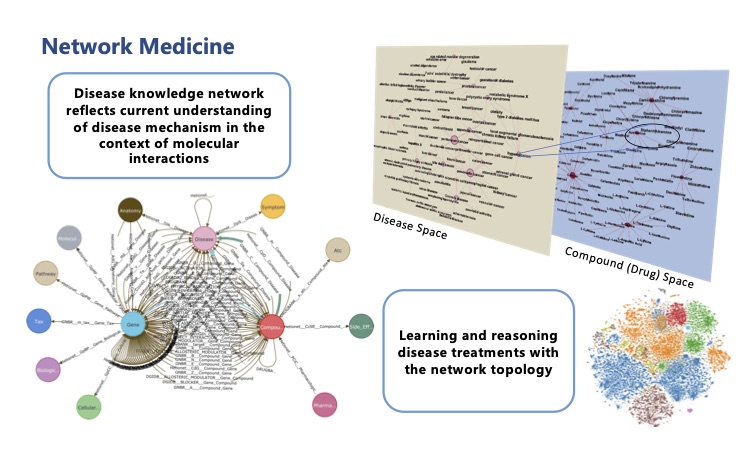
As a preliminary study, we applied state-of-the-art network medicine algorithms on the largest genetic and transcriptomic studies to date in ME/CFS, together with a comprehensive knowledge base we annotated on disease – phenotype (symptoms) – genotype – drug interactions, and computationally predicted drug candidates for the disease.
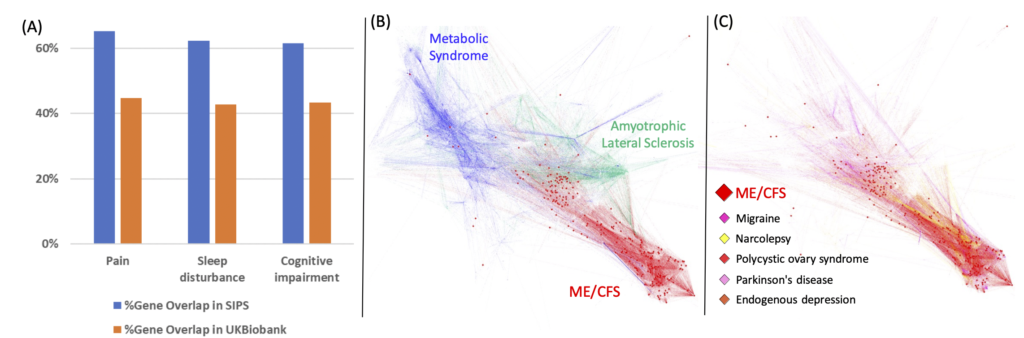
To identify disease gene modules, we will systematically analyze the molecular datasets and associated clinical information of ME/CFS, including genome, epigenome, transcriptome, proteome, cytokine, metabolome, microbiome, clinical lab tests, and clinical symptoms. ME/CFS patients will be clustered into different groups based on their debilitating symptoms, such as fatigue, sleep problems, pain, and sensory disorders, and each group be analyzed separately. For the clinical phenotypes, such as Mast Cell Activation, where molecular data of patients are scarce, we will attempt to use in vitro experimental data in the analysis. We will compare the gene module of ME/CFS and associated clinical symptoms with that of other diseases and symptoms to identify those most similar to ME/CFS. The findings on gene modules of ME/CFS will be reported to the research community for further experimental evaluation.
To identify novel drug candidates, we will integrate the curated information on drugs, diseases, and clinical symptoms with the collected molecular datasets of ME/CFS and related diseases. We will model the molecular signatures of ME/CFS, related clinical symptoms, related diseases, and known drugs, and projected them as well as the various relations into the same semantic space. Both diseases and drugs could be characterized by localized perturbation within a certain neighborhood. The concept is based on the observation that diseases and approved disease-modifying drugs are shown to be projected to close proximity after adding the vector of the relation “treatment” using a pre-trained model in our preliminary analysis. With attention-based algorithms, the weights of neighbors based on known treatments for other similar diseases could be learned and applied to the prediction and reasoning of ME/CFS treatments. The candidate drugs to treat ME/CFS will be identified by the topological closeness of the drugs to ME/CFS and its clinical symptoms after fine-tuning the model for the task of treatment prediction instead of the general link prediction. The direction of the perturbation will be verified with the regulation of the expression of the genes. Results of these candidates will be made available to the research community for experimental verification and clinical study.
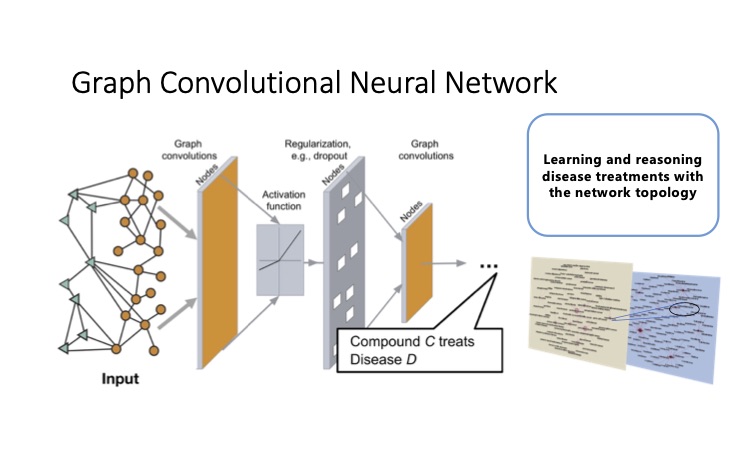
Dr. Chia-Jung Chang is in charge of this project.